Open Data is a very powerful type of asset for people, governments, and commercial entities worldwide. Open datasets, being easy to access, without restrictions to use and process, and interoperable, generate trillions of dollars annually in the global economy. This data, which can be easily crowdsourced and shared, perfectly covers use cases requiring cross-border data collection, transparency, and reliability. Large global markets such as geospatial information systems, human development, and mobility, among others, could not sustain growth without Open Data.
At Skymantics, we have used Open datasets in different ways and forms. From our beloved Open Street Maps which feeds Skymantics Routing Engine, to city mobility datasets that we used in EC Modus, to COPERNICUS fire danger indicators for informing vulnerability-based evacuation, we understand the value Open Data brings when it comes to facilitating research and collaboration.
the limits of open
It is true, however, that Open Data is not a solution for everything. Data-fueled growth in industries of high risk, such as healthcare or financial services, cannot rely on Open Data due to the difficulties in achieving the required levels of accuracy and privacy for strategic business decision-making. In these critical domains, analysis-ready data has value because it contains unique data points, but it is too sensitive to be shared openly. On the other hand, Open datasets are excellent providers of global or statistical truths, but specific inaccuracies or incompleteness must be accepted due to the control of the data capture process and governance being in the hands of a third party.
This is why, when it comes to selecting the data that fuels a specific project or customer development, the innovation and privacy goals need to be well understood in advance:
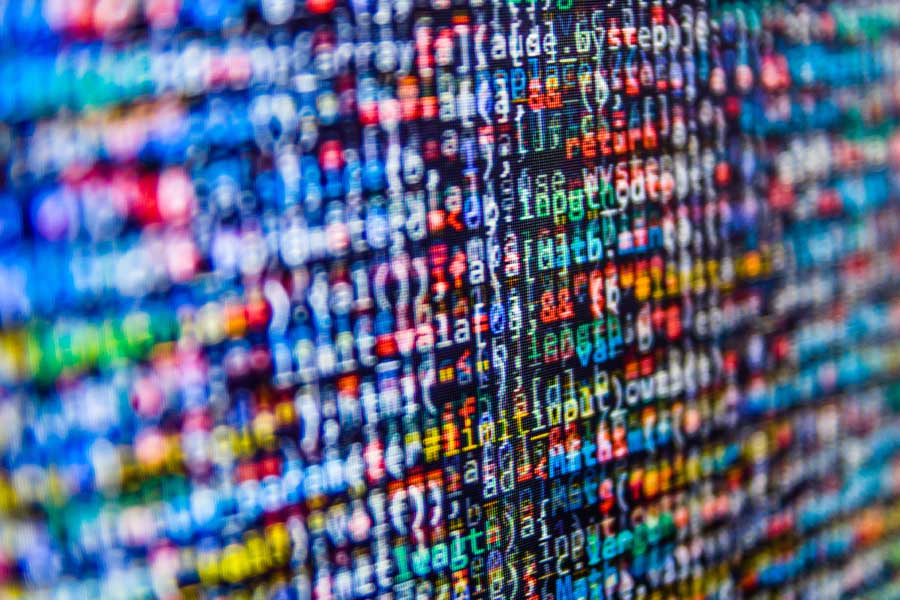
- Is the need related to advancing data application research by means of experimenting with datasets representing well-known geographical or societal variables? If yes, use Open Data.
- Is it instead related to generating enterprise-specific indicators for business decision-making? Then use proprietary data.
An obvious limitation of proprietary data is, well, it is proprietary. If you are the data owner, then it means you are responsible for the curation and governance of such data. If it comes from a partner or is purchased from a data provider, then there are unavoidable costs and barriers for its licensing.
...but What if we use open data to generate synthetic data?
A lesser-known limitation of proprietary data is that datasets captured from real data points (usually called data in production) typically have pieces of information that are too sensitive or confidential, making it difficult or impossible to share. This is a barrier when data analysis results have to be validated by a customer, or if they make part of a collaborative decision-making process involving more than one entity. We have talked in other posts about Skymantics synthetic data solutions as a means to solve this.
However, synthetic data by nature is limited in scope to mimicking data points the enterprise already has. But what if a synthetic dataset is generated, fully or partially, from Open Data samples? That is a very interesting case. I can think of the following benefits of this hybrid approach:
- It provides additional inexpensive, easy to use, source data points used to generate a rich set of attributes which represent accurate depictions of reality as an augmentation to enterprise data sources.
- Since Open Data sources come from authoritative sources, these are certified to be accurate, and are updated in known intervals, lowering the inutility risk
- It increases the transparency of synthetic data, since the sources for validation are readily accessible to anyone. This in turn improves the reputation of synthetic datasets vs being considered “fake data”
We are currently pioneering experiments in doing exactly this – hybridizing Open Data with synthetic data, for example by using U.S. Census source data to generate synthetic population datasets for tax filing analysis. It is a novel area in data research with promising applications where a compromise is sought between accuracy and readiness for innovation. Contact us if you want to know more.